An AI that can play Goat Simulator is a step toward more useful machines
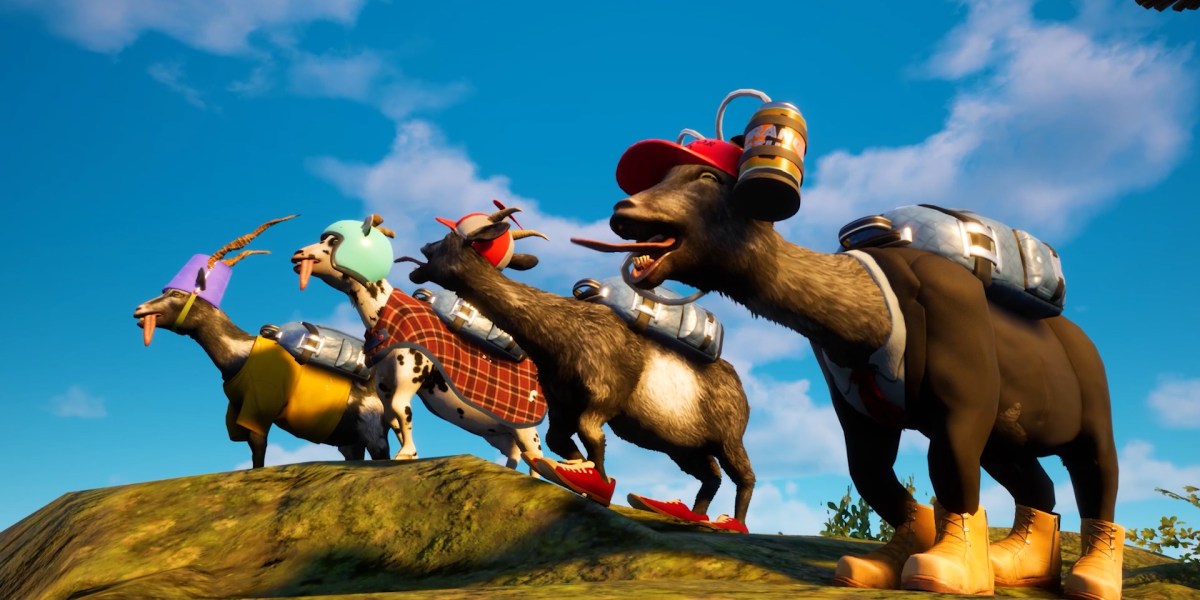
In training AI systems, games are a good proxy for real-world tasks. “A general game-playing agent could, in principle, learn a lot more about how to navigate our world than anything in a single environment ever could,” says Michael Bernstein, an associate professor of computer science at Stanford University, who was not part of the research.
“One could imagine one day rather than having superhuman agents which you play against, we could have agents like SIMA playing alongside you in games with you and with your friends,” says Tim Harley, a research engineer at Google DeepMind who was part of the team that developed the agent.
The team trained SIMA on lots of examples of humans playing video games, both individually and collaboratively, alongside keyboard and mouse input and annotations of what the players did in the game, says Frederic Besse, a research engineer at Google DeepMind.
Then they used an AI technique called imitation learning to teach the agent to play games as humans would. SIMA can follow 600 basic instructions, such as “Turn left,” “Climb the ladder,” and “Open the map,” each of which can be completed in less than approximately 10 seconds.
The team found that a SIMA agent that was trained on many games was better than an agent that learned how to play just one. This is because it was able to take advantage of concepts shared between games to learn better skills and get better at carrying out instructions, says Besse.
“This is again a really exciting key property, as we have an agent that can play games it has never seen before, essentially,” he says.
Seeing this sort of knowledge transfer between games is a significant milestone for AI research, says Paulo Rauber, a lecturer in artificial Intelligence at Queen Mary University of London.
The basic idea of learning to execute instructions on the basis of examples provided by humans could lead to more powerful systems in the future, especially with bigger data sets, Rauber says. SIMA’s relatively limited data set is what is holding back its performance, he says.