Data analytics reveal real business value
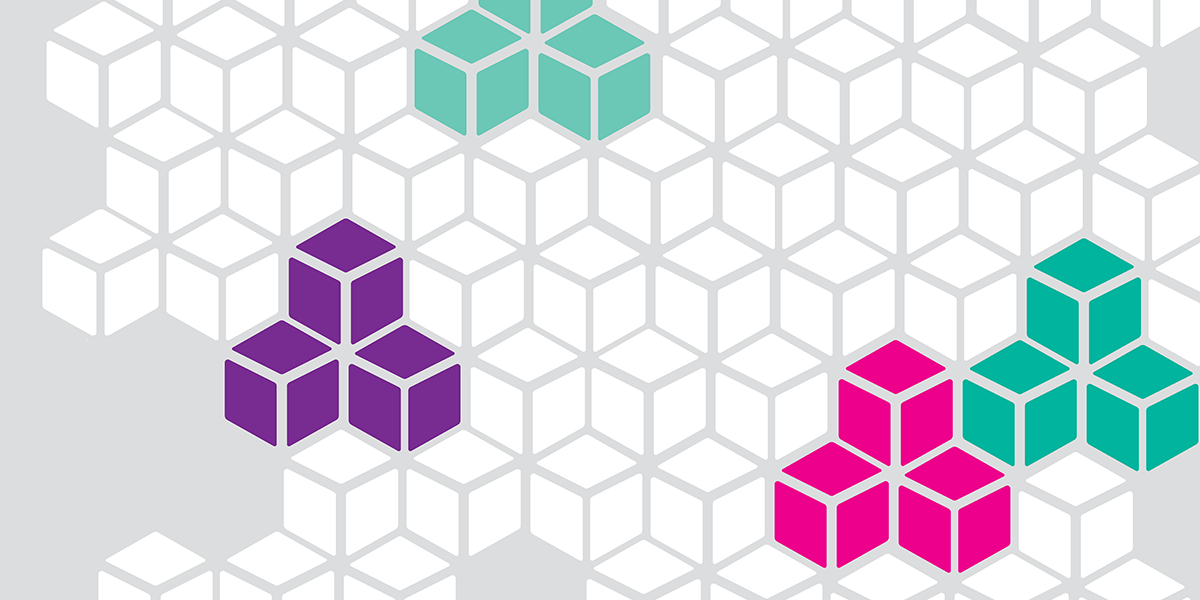
The arrival of enterprise-ready generative AI tools in late 2022 put the need to leverage this data in sharp focus. Given recent months’ enormous hype and heightened expectations around generative AI, having a robust data strategy has become the key imperative for organizations keen to leverage its potential.
Fortunately, data analytics can help organizations identify and extract actionable insights from this underutilized data to support smarter decision-making, streamlined back-office processes, and enhanced business performance. To accomplish this feat, though, business and analytics leaders must ensure data quality while securing the right leadership, employee buy-in, and a data-driven culture.
The benefits of operationalizing data
By 2025, the amount of data in the world will grow to more than 180 zettabytes, according to Statista. This includes the massive streams of data generated by everyday business applications: customer interaction logs, supplier contacts, conversion tracking results, employee and workforce management information, customer feedback data, research results, invoice processing receipts, vendor management. From payroll processing solutions to employee onboarding tools, these technologies produce data whose potential is often underleveraged. That’s changing, however, as organizations turn to data analytics to examine this data, identify patterns, and create models that surface relevant information and recommendations that can lead to more informed decisions.
“Data analytics technology has made huge strides in the last couple of years,” says Sharang Sharma, vice president of business process services at Everest Group. “It’s really phenomenal to see the amount of data that some of these tools can analyze and generate insights from.” In fact, the analytics and business intelligence software market is expected to double in size by 2025, reaching a value of $13 billion, according to Gartner research.

Organizations are already discovering new and innovative ways of operationalizing business data through data analytics. These use cases span industries and demonstrate the power of data analytics to identify inefficient internal processes, particularly back-office workflows, and enhance them for improved business performance.
A grocery store chain, for example, might examine its supply chain data to pinpoint the causes of bottlenecks and delays. Not only do these insights allow the retailer to address delays and act ahead of the curve, but they enable warehouse and procurement managers to optimize inventory in ways that can prevent product waste, customer frustration, and unnecessary costs.
An insurance business might analyze the data generated by human resource management systems to develop new operational insights. Consider, for example, a health insurance company that takes the time to examine data associated with its employee onboarding process. It might identify factors that cause some new hires to take longer than others to become fully productive—and as a result, the business can implement training modules that are designed to boost productivity and minimize turnover. These types of applications are a particular advantage, of course, in highly competitive sectors and in today’s tight labor market.
In a customer support environment, operational efficiencies can be achieved when data analytics tools are used to monitor interaction activity. Certain data patterns may point, for example, to a sudden surge in call volume. Recognizing these patterns can help organizations prepare their staff for upticks and more strategically allocate resources based on fluctuating demand. The result: cost savings, improved customer experience, and new operational efficiencies.
This content was produced by Insights, the custom content arm of MIT Technology Review. It was not written by MIT Technology Review’s editorial staff.