Google DeepMind’s new AI tool helped create more than 700 new materials
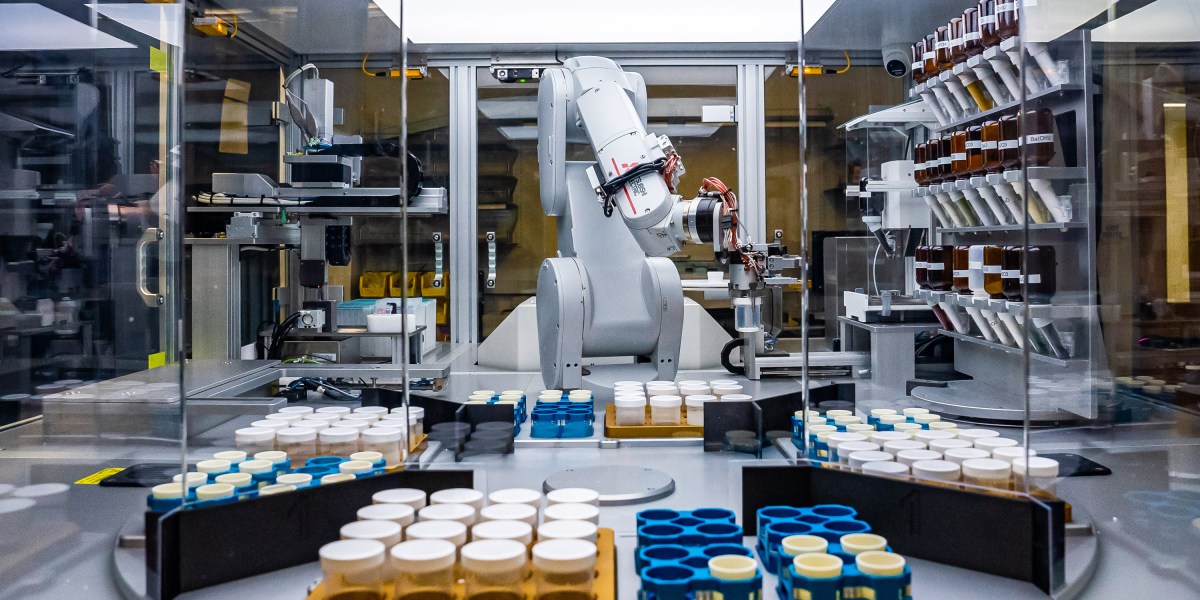
GNoME can be described as AlphaFold for materials discovery, according to Ju Li, a materials science and engineering professor at the Massachusetts Institute of Technology. AlphaFold, a DeepMind AI system announced in 2020, predicts the structures of proteins with high accuracy and has since advanced biological research and drug discovery. Thanks to GNoME, the number of known stable materials has grown almost tenfold, to 421,000.
“While materials play a very critical role in almost any technology, we as humanity know only a few tens of thousands of stable materials,” said Dogus Cubuk, materials discovery lead at Google DeepMind, at a press briefing.
To discover new materials, scientists combine elements across the periodic table. But because there are so many combinations, it’s inefficient to do this process blindly. Instead, researchers build upon existing structures, making small tweaks in the hope of discovering new combinations that hold potential. However, this painstaking process is still very time consuming. Also, because it builds on existing structures, it limits the potential for unexpected discoveries.
To overcome these limitations, DeepMind combines two different deep-learning models. The first generates more than a billion structures by making modifications to elements in existing materials. The second, however, ignores existing structures and predicts the stability of new materials purely on the basis of chemical formulas. The combination of these two models allows for a much broader range of possibilities.
Once the candidate structures are generated, they are filtered through DeepMind’s GNoME models. The models predict the decomposition energy of a given structure, which is an important indicator of how stable the material can be. “Stable” materials do not easily decompose, which is important for engineering purposes. GNoME selects the most promising candidates, which go through further evaluation based on known theoretical frameworks.
This process is then repeated multiple times, with each discovery incorporated into the next round of training.
In its first round, GNoME predicted different materials’ stability with a precision of around 5%, but it increased quickly throughout the iterative learning process. The final results showed GNoME managed to predict the stability of structures over 80% of the time for the first model and 33% for the second.